Box Blur Algorithm – With Python implementation
Last Updated :
30 Dec, 2020
The pixels in an image are represented as integers. After blurring each pixel ‘x’ of the resulting image has a value equal to the average of the pixels surrounding ‘x’ including ‘x’. For example, consider a 3 * 3 image as
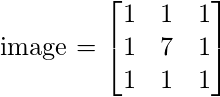
Then, the resulting image after blur is blurred_image =

So, the pixel of blurred image is calculated as (1 + 1 + 1 + 1 + 7 + 1 + 1 + 1 + 1) / 9 = 1.66666 = 1
Box Blur Algorithm –
Box blur is also known as box linear filter. Box blurs are frequently used to approximate Gaussian blur.
A box blur is generally implemented as an image effect that affects the whole screen. The blurred colour of the current pixel is the average of the current pixel’s colour and its 8 neighbouring pixels.
Note: For each 3 * 3 pixel matrix there is one blurred pixel calculated as above.
FOr Example, Consider the below image.
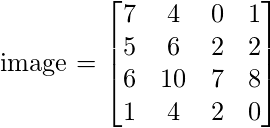
It’s blurred image is given below:
Explanation:
There are four 3 * 3 matrix possible in the above image. So there are 4 blurred pixel in the resulting image. The four matrices are:
,
,
,
and
Implementation in Python:
def square_matrix(square):
tot_sum = 0
for i in range ( 3 ):
for j in range ( 3 ):
tot_sum + = square[i][j]
return tot_sum / / 9
def boxBlur(image):
square = []
square_row = []
blur_row = []
blur_img = []
n_rows = len (image)
n_col = len (image[ 0 ])
rp, cp = 0 , 0
while rp < = n_rows - 3 :
while cp < = n_col - 3 :
for i in range (rp, rp + 3 ):
for j in range (cp, cp + 3 ):
square_row.append(image[i][j])
square.append(square_row)
square_row = []
blur_row.append(square_matrix(square))
square = []
cp = cp + 1
blur_img.append(blur_row)
blur_row = []
rp = rp + 1
cp = 0
return blur_img
image = [[ 7 , 4 , 0 , 1 ],
[ 5 , 6 , 2 , 2 ],
[ 6 , 10 , 7 , 8 ],
[ 1 , 4 , 2 , 0 ]]
print (boxBlur(image))
|
Output:
[[5, 4],
[4, 4]]
Test case 2:
image = [[ 36 , 0 , 18 , 9 ],
[ 27 , 54 , 9 , 0 ],
[ 81 , 63 , 72 , 45 ]]
print (boxBlur(image))
|
Output:
[[40, 30]]
Further Read: Box Blur using PIL library | Python
Please Login to comment...